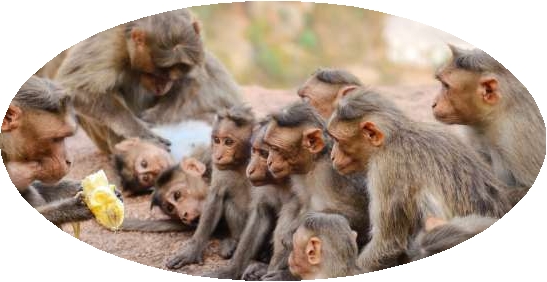
Some time back, while tinkering with R, I coded up a version of the Marascuilo procedure and wrote up the results in a post to my old blog, which I am now resurrecting here. As you probably know, the Marascuilo procedure is used to analyze the difference between two proportions in a contingency table to determine if that difference in proportion is significant or not. The function I wrote will take every possible pairwise combination and print Y(es) or N(o) to indicate whether or not the differences in proportions are statistically significant.
For a real world-ish use case, imagine you are managing three hotels: the Grand Plaza (GP), Plaza Royale (PR), and the Plaza Prima (PP). One determinant of service quality at your hotels is the presence or absence of vermin (insects, rodents, etc.) Your staff has conducted a survey of guests at all three hotels in which they were asked, “were you bothered by any vermin during your stay?” You decide to use the Marascuilo Procedure to determine if any one (or more) hotels is/are significantly under-performing other hotels in the ‘infested with vermin’ category.
The R commands below show the frequency table that captures the survey responses, and shows how we would invoke our marascuilo function to determine which (if any) hotel’s performance is significantly different from the others. Note that our function outputs the results of three pairwise combinations. This is correct because we have three items and there 3 choose 2, i.e., three, ways to pick two items from a list of three.
> lines <- "
+ GP PR PP
+ Y 128 199 126
+ N 88 33 66
+ "
>
> con <- textConnection(lines)
> tablefoo <- read.table(con, header=TRUE)
> close(con)
>
>
> marascuilo(tablefoo)
pair abs.diff critical.range significant
[1,] "GP | PR" "0.265166028097063" "0.0992354018215412" "Y"
[2,] "GP | PP" "0.0636574074074074" "0.117201905174372" "N"
[3,] "PR | PP" "0.201508620689655" "0.100947721261772" "Y"
The results indicate no significant difference between the performance of the Grand Plaza (GP) and the Plaza Prima (PP) on the metric in question. However both the Grand Plaza and the Plaza Prima (PP) are shown to differ significantly from the Plaza Royale (PR). The Plaza Royale guest’s proportion of ‘yes’ responses to the vermin question was the highest of the three hotels. Therefore, if you decide to take any action to address this problem, you should probably start with the Plaza Prima. (A flame thrower might help.)
The Code
# marascuilo -
#
# Perform the Marascuilo procedure on all pairwise combinations of
# proportion differences from a contingency table to see which one
# (if any) is significant.
#
# Arguments are:
#
# dataFrame:
# a data.frame with named rows and columns. The
# names of the groups being compared are assumed to be the columns.
#
# confidence:
# the degree of confidence with which to estimate the chi squared constant.
# the default is .95.
#
marascuilo = function(dataFrame,confidence=.95) {
chiResult = chisq.test (dataFrame, correct=FALSE )
xSquared = chiResult$statistic
# Generate all possible pair-wise combinations of groups
colNames = names(dataFrame)
combos = combn(colNames , 2)
numCombos = dim(combos)[2] # combos is an array of pairs, we want the length
# Allocate matrix (initially 0 rows) for results
results = matrix(nrow=0, ncol=5, dimnames=getResultsColumNames() )
chiSquaredConstant = calcChiSquaredConstant(dataFrame, confidence)
for (i in 1: numCombos) {
newRow = testSignificanceOfAbsDiffVsCriticalRange(
dataFrame, combos, i, chiSquaredConstant )
results = rbind(results, newRow) # append new row to results
}
# sort results so that the pair differences that most strikingly exceed
# the critical range appear toward the top.
sortedResults = results[ order( results[,'abs.diff-critical.range'] ) , ]
return (sortedResults )
}
calcChiSquaredConstant = function(dataFrame,confidence) {
nRows = dim(dataFrame)[1]
nCols = dim(dataFrame)[2]
degreesFreedom = (nRows-1) * (nCols-1)
chiSquaredConstant = sqrt( qchisq(confidence,degreesFreedom) )
return (chiSquaredConstant)
}
getResultsColumNames = function (numRows) {
return (
list(
c(),
c('pair', 'abs.diff', 'critical.range', 'abs.diff-critical.range', 'significant')
)
)
}
# test significance for ith combination
#
testSignificanceOfAbsDiffVsCriticalRange = function(
dataFrame, combos, i, chiSquaredConstant) {
results = matrix(nrow=1, ncol=5, dimnames=getResultsColumNames() )
pair1=combos[1,i]
pair2=combos[2,i]
# sum column denoted by name 'pair1' into groupTotal1
groupTotal1 = sum( dataFrame[ , pair1])
groupTotal2 = sum( dataFrame[ , pair2]) # do same thing for pair2...
p1 = dataFrame[1, pair1] / groupTotal1
p2 = dataFrame[1, pair2] / groupTotal2
p1Not = (1 - p1)
p2Not = (1 - p2)
absDiff = abs( p2 - p1 )
criticalRange = chiSquaredConstant *
sqrt(p1*p1Not/groupTotal1 + p2*p2Not/groupTotal2)
results[1, 'pair'] = paste(pair1,"|",pair2)
results[1, 'abs.diff'] = round(absDiff,3)
results[1, 'critical.range'] = round(criticalRange ,3)
results[1, 'abs.diff-critical.range'] = round(absDiff - criticalRange ,3)
if (absDiff > criticalRange) {
results[1, 'significant'] = 'Y'
} else {
results[1, 'significant'] = 'N'
}
return(results)
}