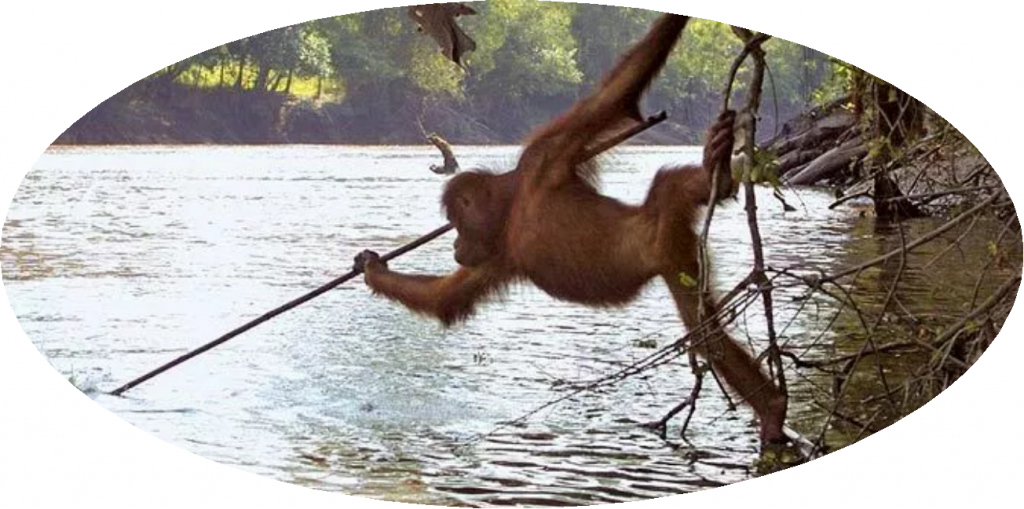
I recently went looking for a good Docker recipe to locally launch a Spark 2.4.3 cluster in stand-alone mode. Running in ‘local’ mode is good for roughing out your business logic and unit tests, but it will not flush out bugs that only surface in a fully distributed environment. That is where integration tests come in, and while some organizations will set up a test cluster for this purpose, you don’t want to be twiddling your thumbs when your network is down, or your admin decides to take down the test cluster you depend on for maintenance. This is where Docker comes to our rescue.
This project contains a fairly recent (2.2.0) Dockerfile and docker-compose.yml that will bring up a multi-node stand-alone Spark cluster, but I wanted 2.4.3. So, I forked the project and brought it up to date, plus I added a little example project to make it easy to test out.
Below I lay out the steps you can follow to get a stand-alone cluster up and running on whatever machine you use (provided you have git, docker and docker-compose already installed). Three caveats: (1) the docker-compose.yml is set to version “2” and if you use a later version than me, you might need to set it to “3”, (2) this was tested on Linux, but I am very sure that Docker commands on Mac will work the same — not at all sure about Windows, (3) I am assuming you have the proper version of Scala for Spark 2.4.3 installed (2.12.x) on your machine, and that you have downloaded Spark 2.4.3 locally on your machine to run spark-submit.
Getting The Cluster Up In Your Environment
Open two terminals, in each one cd to /tmp. In the first, type:
git clone git@github.com:buildlackey/docker-spark-standalone.git
cd docker-spark-standalone
docker-compose up
You will see logs for the client (client_1), master (master_1), and worker (worker_1) nodes of the cluster. Don’t worry if you see
Failed to connect to master/172.24.0.4:7077
in the worker_1 log at the start of the boot process. The worker is trying to connect to a master which is not fully up. This will work itself out, and in 5 seconds or so you should see:
master_1 | 19/08/25 02:56:35 INFO Master: Registering worker 172.24.0.2:36655 with 4 cores, 4.0 GB RAM
worker_1 | 19/08/25 02:56:35 INFO Worker: Successfully registered with master spark://master:7077
Now, in the second window type:
cd /tmp/docker-spark-standalone/spark-example
sbt package
This will create the .jar file you will submit to spark as follows:
spark-submit --master spark://127.0.0.1:7077 --class SimpleApp \
--name simple target/scala-2.12/simple-project_2.12-1.0.jar
You should then see output that looks something like this:
2019-08-24 20:08:25 WARN Utils:66 - Your hostname, chris-laptop resolves to a loopback address: 127.0.1.1; using 192.168.1.83 instead (on interface wlp4s0)
2019-08-24 20:08:25 WARN Utils:66 - Set SPARK_LOCAL_IP if you need to bind to another address
2019-08-24 20:08:25 WARN NativeCodeLoader:62 - Unable to load native-hadoop library for your platform... using builtin-java classes where applicable
2019-08-24 20:08:25 INFO SparkContext:54 - Running Spark version 2.3.1
2019-08-24 20:08:25 INFO SparkContext:54 - Submitted application: Simple Application
..... Lots more junk...
2019-08-24 20:08:30 INFO CodeGenerator:54 - Code generated in 12.991607 ms
+-----+
|value|
+-----+
|hi ho|
+-----+
No luck Submitting From My IDE.
After importing the sample project into Intellij I thought there would be no problem running it via right click. But regretably, that was not my fate. I am continuing to see the error below when I run locally, which is really irksome.
java.io.InvalidClassException: org.apache.spark.rpc.netty.NettyRpcEndpointRef; local class incompatible:
If I figure this out I will update this post.